Understanding Predictive Intelligence: Key Concepts and Technologies Explained
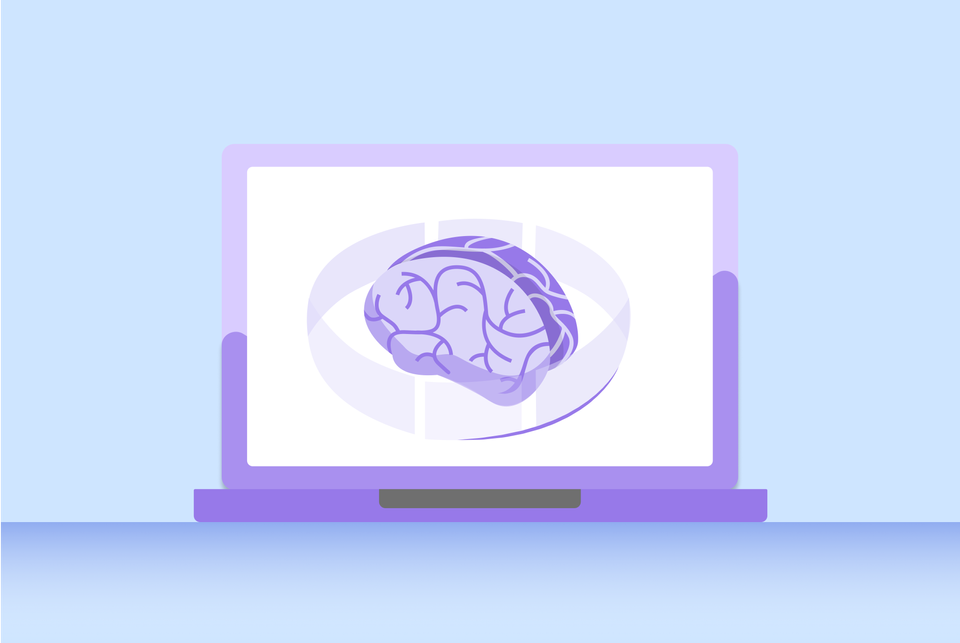
In today's competitive business landscape, businesses increasingly turn to data-driven solutions to enhance their sales strategies. Predictive Intelligence, a powerful analytical approach, leverages historical and real-time data to forecast future outcomes, enabling businesses to make informed decisions.
What is Predictive Intelligence?
Predictive Intelligence refers to the use of advanced data analytics, machine learning algorithms, and statistical techniques to analyze historical data and make predictions about future events or behaviors. Unlike traditional analytics, which describes past performance, Predictive Intelligence forecasts potential outcomes, enabling organizations to proactively address challenges and seize opportunities.
Predictive Intelligence allows sales teams to personalize their outreach by adapting communications to the unique traits and behaviors of each lead. This customized strategy improves engagement and enhances the likelihood of converting.
What type of data does Predictive Intelligence need?
Combining three types of data allows businesses to build more accurate predictive models, leading to better decision-making and improved outcomes. For effective predictive intelligence, three types of data are essential:
- Historical Data: This includes records and interactions, such as sales figures, customer behaviors, marketing campaign performance, and operational metrics. Historical data provides the foundation for identifying patterns and trends that predictive models use to forecast future outcomes.
- Real-Time Data: This type of data is continuously collected and reflects the current state of the business environment. Examples include website traffic, social media interactions, and customer inquiries. Real-time data helps organizations respond quickly to changes and refine predictions based on the most up-to-date information.
- Contextual Data: This encompasses external factors that can influence predictions, such as market trends, economic indicators, and competitive intelligence. Contextual data enriches the analysis by providing additional insights.
Applications of Predictive Intelligence
Predictive Intelligence has a wide range of applications across various industries. In marketing, businesses can anticipate customer behavior, segment audiences, and optimize campaigns for improved targeting and conversion rates. In finance, institutions utilize predictive models to assess credit risk, detect fraud, and forecast market trends. Healthcare providers benefit from predictive analytics in patient care management, predicting disease outbreaks, and enhancing operational efficiency. Meanwhile, retailers leverage predictive intelligence for effective inventory management, demand forecasting, and creating personalized customer experiences. This versatility makes predictive intelligence a valuable tool for organizations looking to drive better outcomes in their respective fields.
Technologies Behind Predictive Intelligence
- Machine Learning: Machine learning algorithms are fundamental to predictive intelligence. They enable systems to learn from data and improve their predictions without being explicitly programmed. Common algorithms include supervised learning methods and unsupervised learning methods.
- Big Data Technologies: The volume and variety of data today require robust technologies for storage and processing.
- Data Visualization Tools: Visualizing predictive insights is crucial for decision-makers. Tools like Google Data Studio help transform complex data into clear, actionable insights through interactive dashboards and visual representations.
- Cloud Computing: Cloud platforms provide scalable resources for data storage and processing, making it easier for organizations to leverage predictive intelligence without the need for extensive on-premises infrastructure. Services like AWS, Google Cloud, and Microsoft Azure offer powerful analytics tools that can handle large-scale data processing.
- Natural Language Processing (NLP): NLP technologies enable the analysis of unstructured data, such as customer reviews or social media interactions. By extracting sentiment and context from text, organizations can gain deeper insights into customer behavior and preferences.
Conclusion
Predictive Intelligence is revolutionizing how organizations make decisions by providing insights that anticipate future trends and behaviors. By understanding the key concepts and technologies involved, businesses can harness the power of predictive analytics to drive innovation, improve customer experiences, and achieve a competitive edge in their respective markets. As technology continues to advance, the potential for Predictive Intelligence will only expand, making it an essential component of modern business strategy.
Use the power of predictive intelligence and improve your lead generation using strong, data-driven technologies. Unlock new growth opportunities and discover what DeepSales can do for you!